Don't fear the bot!
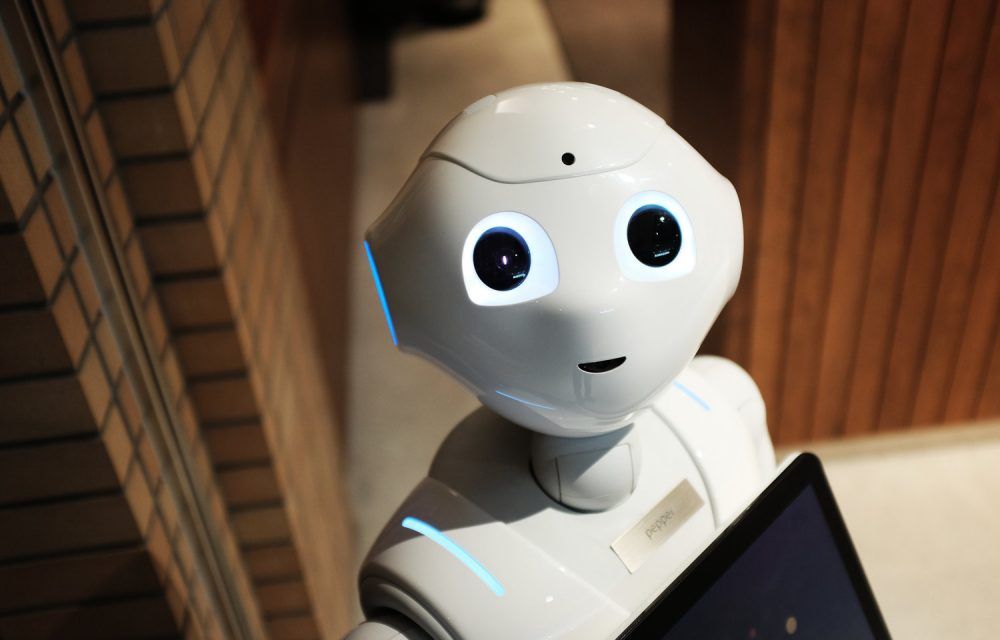
In the infamous words of Benjamin Franklin, “In this world, nothing can be said to be certain except death, taxes [and more data]”. Today’s world is awash with data, which, if used wisely, can uncover meaningful information, drive business predictions and enhance decision-making. As data has drifted into the mainstream over the last couple of decades, new roles, including business intelligence analyst, data visualisation specialist and data scientist, to name a few, have become fundamental to organisations. All were created to mine the value lurking in business data from customers, products, advertising and social media sources.
Such is the opportunity to get ahead of competitors by leveraging data. The Economist published this article in 2017 naming data as the oil of the digital era. But unlike oil, the availability of data that can be consumed looks set to only increase with each passing day.
Mo Data, Mo Problems
My first foray into the data analytics world began at a digital media start-up where I was quickly tasked with the responsibility of mining the data for insights and reporting on the overall health of the business. It was exciting to watch the data reporting processes evolve from the typical Excel, CSV and PDF formats to the use of interactive and self-service analytics dashboards. As the value of data became more apparent, so did the demand from the editorial, marketing, subscriptions, ad operations and executive teams to utilise their data. My to-do list soon felt like a never-ending landslide of requests for ad-hoc analysis and routine reports, which at times made me feel like the bottleneck to the progress of the business. My experience there was enlightening and quickly made me aware of some valuable lessons:
Data is fragmented, making fully-formed insights difficult Like many fast-growing businesses, we were no different in that the data from the various departments was stored in silos with no central database to query. In addition to my laptop becoming a dumping ground for CSV files, it made it increasingly challenging for me to understand why certain trends were occurring. I would often see a decline in eCPMs on a given day without knowing that the cause was a faulty ad unit due to a code update on the site by the dev team. It would be easy to chalk that day off as just a bad day for ad spend instead.
Yet another dashboard that gets no love. With the increase of requests for analysis, I soon found that I was making my dashboards with tons of filters to try and please every stakeholder, which led to no one finding that the dashboard fitted their exact needs. Furthermore, it was disheartening to see yet another dashboard I had spent hours trying to make user friendly and engaging receive hardly any views after its initial deployment while still getting questions in my inbox, which the dashboard already had the answers to.
Cleaning data and writing reports take forever! As exciting as this new world of being a data scientist sounds, the reality is that analysts spend the majority of their time cleaning and transforming their data into a format that can be read for analysis. Once you have finally taken a deep dive into your insights, then comes the crucial stage of communicating it effectively. My Mondays were typically filled with weekly performance reviews and crafting each email would easily take me close to an hour or more. To compound things, it was always frustrating when I saw I had left in a number or forgot to change a data point in my edits from the previous version of the report.
Never fear, the AI is here! The Knowledge Project With Shane Parrish podcast episode with AI expert Pedro Domingos discussed “The Rise of The Machines” and tackled an interesting topic about how white collar jobs are easier to replace blue collar jobs. For many current and former analysts like myself, that may sound like a major threat to our livelihoods, but I would highly recommend giving it a listen. It covers the important concept of how AI can be used to empower human workers rather than replace them.
It was in this vein that my co-founders and I decided to start Spiny.ai. Our smart Publisher Intelligence (PI) sidekick, nicknamed Pliny the Editor, enables publishing business to create data-conscious organisations by empowering each individual to become a data storyteller within their own function. Our experience in the publishing industry taught us the value of storytelling and the timely delivery of pieces. Building on that knowledge and through the use of natural language generation (NLG) techniques, Pliny creates narratives from data and delivers it in a quick easy-to-digest, bitesize fashion.
As we’ve deemed it important with all new emerging technologies, our PI keeps humans in the loop by enabling them to craft their own stories through selecting the metrics they are most interested in and being able to add more context to Pliny’s automatically generated data stories. As such, the way organisations report their data and insights should be narrative-driven. Pliny accelerates this transition by increasing the speed, contextualisation and tweet-like delivery of the insights.
How Pliny can make analysts (and non-data employees) even better at their role:
- As previously mentioned, a large majority of the time, data needs to be retrieved, cleaned and transformed before any insights can be gleaned. Pliny automates this process, finding the right insights that need to be investigated from the various streams of data and automatically begins generating the story. With the predictable side of the analyst role being automated, this leaves you more time to actually dig for meaningful insights or investigate what Pliny has already found for you.
- Stakeholders engaged far more when I built narratives around the trends visualised in my dashboards in a story-like format. The key distinction was that dashboards lacked the emotions that stories can convey, which are an essential part of the decision making process. With Pliny creating bite-size stories into a personalised timeline for each team, analysts can focus more on how they communicate these insights to different teams. Discovering that 60% of all sessions are from Facebook in a given month may seem like great news to the marketing team but not so great for the ad ops team who knows that Facebook sessions do not monetise as well as others on their site.
- The data analyst role is set to evolve into the data coach. Brent Dykes wrote a great piece for Forbes describing this concept. As data becomes democratised, the burden of business intelligence becomes a shared one, no longer resting solely on the shoulders of the business intelligence and data teams. Pliny’s format of creating bite-size data stories that can be consumed in a similar fashion to how we consume our Twitter, Apple News and Google News feeds creates a subconscious of data awareness in the business. With each member having the ability to understand data, the role of the analyst will be to help coach others to tell data stories and make impactful decisions, with Pliny in tow, aiding in the writing of these stories.
With data now consumable by everyone in a centralised communication sphere, trust improves and the biases that can accompany data reports are mitigated. Better yet, each team member is focusing more on what actually drives value to their teams rather than spending time trying to answer head-scratching questions.
Ready to Get Started?
See how Spiny can put boosting your revenue on autopilot